Using Predictive Analytics for Home Prices
- Jul 31, 2023
- 4 min read
According to data from the U.S. News Housing Market Index, over 4 million houses will be bought and sold in 2023. Whether buying a house or selling one, everyone wants to ensure they are getting a fair deal. But, the housing market can be unpredictable, making it difficult to predict the price of a home. However, data science has proven one effective way to predict home prices and provide essential information to buyers and sellers.
The Zestimate
Zillow is the most widely used real estate website in the United States. One tool this site offers to visitors is the “Zestimate,” a prediction of a house’s price and monthly rental income. While Zillow keeps the specifics of this data analysis tool under wraps, it’s proven an accurate method. It has a median error of 2.35% for active listings. This error is calculated by comparing a Zestimate to a house’s final sale price.
As mentioned, the exact techniques Zillow uses to create and update the Zestimate are unknown. However, Zillow does acknowledge that the Zestimate uses comparable sales close to the house as well as physical attributes of the house, historical data, and other on-market data. While comparable sales are certainly an essential feature to consider when predicting the price of a house, this does introduce a drawback to Zestimate’s predictions. Areas with fewer house sales are likely to have less accurate results than areas with more home sales due to the decreased availability of data.
Other Predictive Analysis Methods
While the Zestimate is a popular predictive analysis tool in the housing market, it is only one such example. Several other teams have created tools for predicting housing prices by using a variety of machine learning algorithms.
One such team sought to predict the housing price for buildings in Beijing. The team began with a dataset of 300,000 houses. The team then cleaned the data by removing missing data and determining the most applicable attributes of the data. One thing the team noted was the strong correlation between the district a house was in and the price of the house, as shown in the graph to the right.

After cleaning the data, three machine learning algorithms were trained: Random Forest, Extreme Gradient Boosting (XGBoost), Light Gradient Boosting Machine (LightGMB), Hybrid Regression, and Stacked Generalization. After training each algorithm on the testing data, its accuracy was validated on a test dataset that was withheld from the training data. While the Random Forest algorithm proved most accurate on the training data, the stacked Generalization Regression algorithm was most accurate on the testing data. This suggests the Random Forest algorithm created a model that was overfitted.
Machine learning has also been used to predict house prices in India. A team from the Vidyalankar Institute of Technology used several different machine learning algorithms to predict home prices in Bangalore. Before training the algorithms, the team used a correlation matrix to analyze the data. Like the previous team, they discovered a high correlation between several different attributes in the dataset. The correlation matrix is shown below. The darker the color, the stronger the positive correlation. The lighter the color, the more negative the correlation.
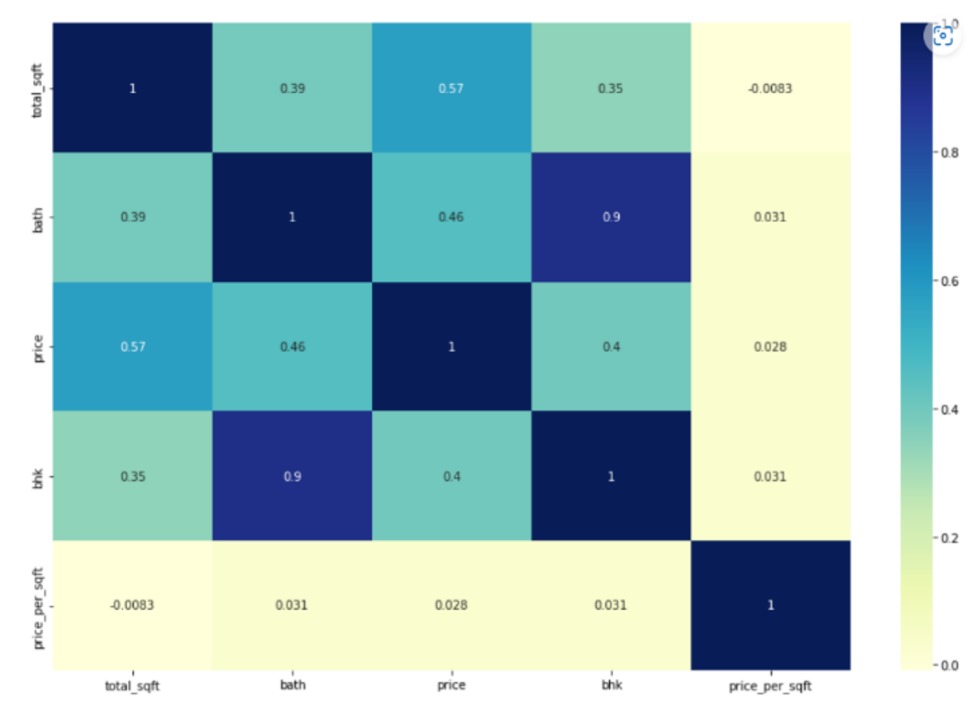
As you can see from the graph, there is a high positive correlation between the number of baths and bhk (an Indian abbreviation for bedroom hall kitchen).
After exploring the correlation between data points, the team trained a Linear Regression, Least Absolute Shrinkage and Selection Operator (LASSO), and a Decision Tree algorithm. In this case, Linear Regression proved the most accurate algorithm, while LASSO had the
worst performance.
Conclusion
While the Zestimate is a common prediction method for house prices, it is not without its downsides. Since the tool relies on house sales to create predictions, areas with fewer house sales are likely to have less accurate predictions. However, the Zestimate is not the only prediction method available. Teams from around the world have used a variety of machine learning algorithms to predict housing prices. While each team used a different approach, they both showcased key considerations when using predictive analysis.
Both teams started their analysis by understanding their data. Box and whisker plots and correlation matrices are effective ways of visualizing the correlation between an attribute and the predicted value and the correlation between attributes, respectively. When it comes to choosing a machine learning algorithm, train several algorithms and evaluate their accuracy against a testing data set. Based on each trained algorithm’s performance, the most accurate algorithm can be chosen to create predictions. Both teams determined a regression algorithm created the most accurate predictions, making regression algorithms an excellent starting point for similar predictive problems.
With over four million houses likely to be sold this year, many people will turn to predictive analysis methods to determine if they are getting a fair deal or not. These tools can provide buyers and sellers with peace of mind during their home-buying process. But it’s important to remember that any predictive analysis tool is just that, a tool. At the end of the day, a house is worth whatever someone is willing to pay for it.
Opmerkingen